Overview
The diversity of backgrounds and research interests in our group is also reflected in the wide range of technologies that we use and develop. The vignettes below provide an overview of tools that were developed by us. Particular areas of expertise are network-based methods, Virtual Reality technology, multi-omics data integration and image analysis. More information on specific projects, links to code and webapps can be found under resources.
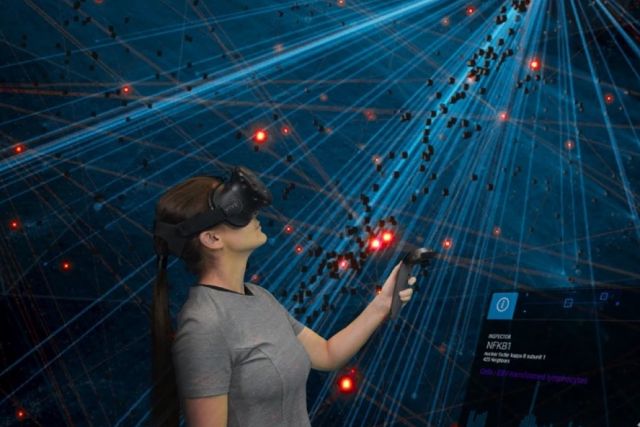
Our team is pioneering the application of Virtual Reality (VR) technology for exploring large and diverse biological data. On the surface, VR simply drastically increases the amount of information that can be displayed. On a deeper level, and perhaps more importantly, the immersive 3D space also represents the natural environment in which evolution has shaped human cognition. We perceive and interact with the world in 3D, and basic neurological processes of pattern recognition, learning, even social behavior are intimately linked to this fundamental experience. Exploring data in VR thus offers unique opportunities for integrating powerful machine learning with innately human capabilities, e.g. intuition and generalization via experience, incomplete or noisy information.
NATURE COMMUNICATIONS (2021)
doi.org/10.1038/s41467-021-22570-w [pdf]
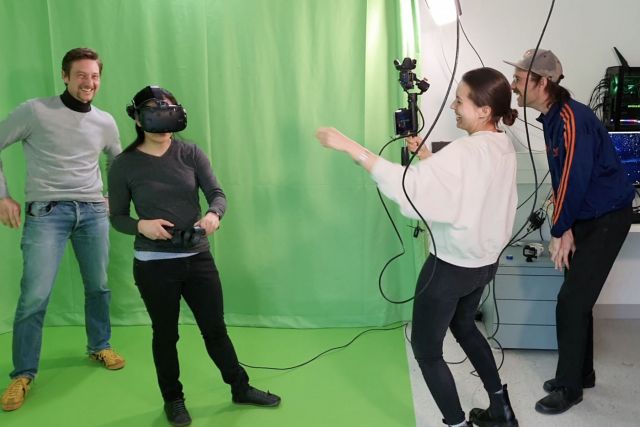
We built a multi-media workshop as a central infrastructure for developing and experimenting with new technologies for exploring data. The room is equipped with a range of state-of-the-art 3D technologies. Several Virtual Reality stations enable several users to simultaneously and collaboratively explore a complex dataset in a shared virtual environment. The green screen and custom-built video and audio recording equipment allow us to create mixed reality videos and explore new formats for science communication and remote teaching.
Example videos produced in our multi-media kitchen.
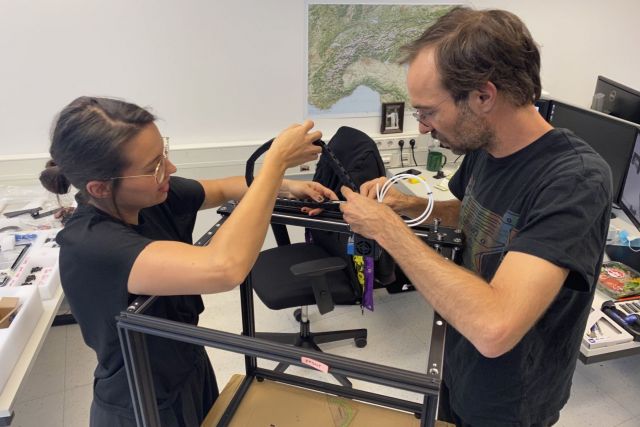
In addition to our multi-media kitchen, we also set up a creative workshop for producing physical objects. The workshop is equipped with a 3D printer, laser cutter, electronic workbench, and other tools. We use the workshop to develop experimental user interfaces for virtual, augmented and mixed reality (VR/AR/XR) applications, for example data gloves or AR panels, but also to produce a wide variety of physical artifacts, for example 3D protein models, and art pieces.
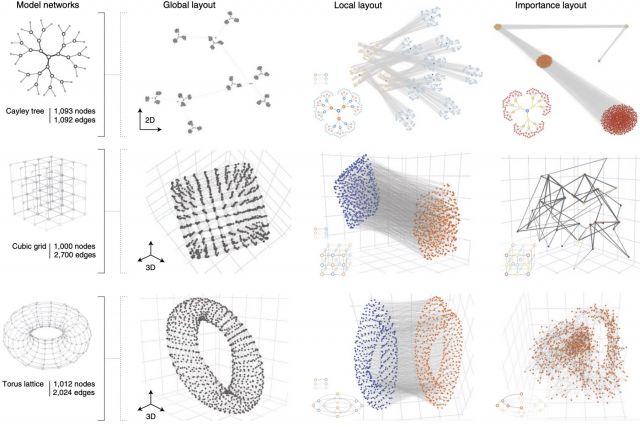
Networks offer an intuitive visual representation of complex systems. Important network characteristics can often be recognized by eye and, in turn, patterns that stand out visually often have a meaningful interpretation. In conventional network layout algorithms, however, the precise determinants of a node’s position within a layout are difficult to decipher and to control. Here we propose an approach for directly encoding arbitrary structural or functional network characteristics into node positions. We introduce a series of two- and three-dimensional layouts, benchmark their efficiency for model networks, and demonstrate their power for elucidating structure-to-function relationships in large-scale biological networks.
NATURE COMPUTATIONAL SCIENCE (2022)
doi.org/10.1038/s43588-022-00199-z [pdf]
NATURE RESEARCH BRIEFING (2022)
doi.org/10.1038/s43588-022-00203-6 [pdf]
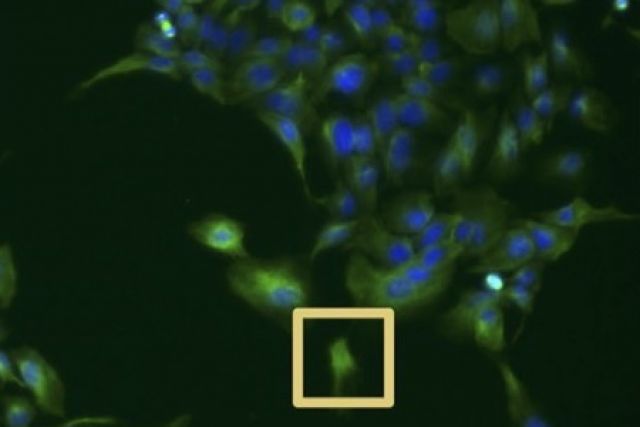
High-content imaging screens provide a cost-effective and scalable way to assess cell states across diverse experimental conditions. The analysis of the acquired microscopy images involves assembling and curating raw cellular measurements into morphological profiles suitable for testing biological hypotheses. Despite being a critical step, general-purpose and adaptable tools for morphological profiling are lacking and no solution is available for the high-performance Julia programming language. Here, we introduce BioProfiling.jl, an efficient end-to-end solution for compiling and filtering informative morphological profiles in Julia. The package contains all the necessary data structures to curate morphological measurements and helper functions to transform, normalize and visualize profiles. Robust statistical distances and permutation tests enable quantification of the significance of the observed changes despite the high fraction of outliers inherent to high-content screens. This package also simplifies visual artifact diagnostics, thus streamlining a bottleneck of morphological analyses.
BIOINFORMATICS (2021)
doi.org/10.1093/bioinformatics/btab853 [pdf]
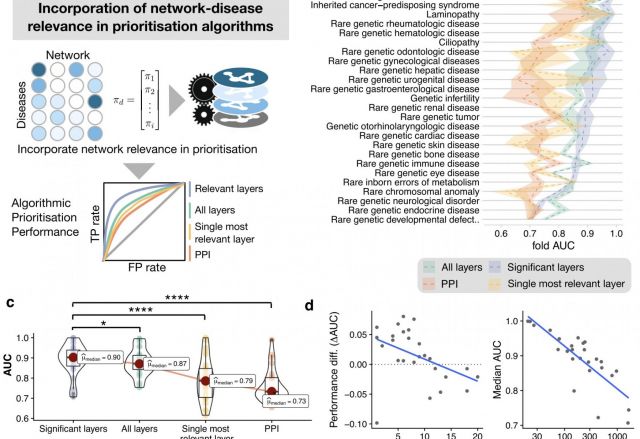
Rare genetic diseases are typically caused by a single gene defect. Despite this clear causal relationship between genotype and phenotype, identifying the pathobiological mechanisms at various levels of biological organization remains a practical and conceptual challenge. Here, we introduce a network approach for evaluating the impact of rare gene defects across biological scales. We construct a multiplex network consisting of over 20 million gene relationships that are organized into 46 network layers spanning six major biological scales between genotype and phenotype. A comprehensive analysis of 3,771 rare diseases reveals distinct phenotypic modules within individual layers. These modules can be exploited to mechanistically dissect the impact of gene defects and accurately predict rare disease gene candidates. Our results show that the disease module formalism can be applied to rare diseases and generalized beyond physical interaction networks. These findings open up new venues to apply network-based tools for cross-scale data integration.
NATURE COMMUNICATIONS (2021)
doi.org/10.1038/s41467-021-26674-1 [pdf]
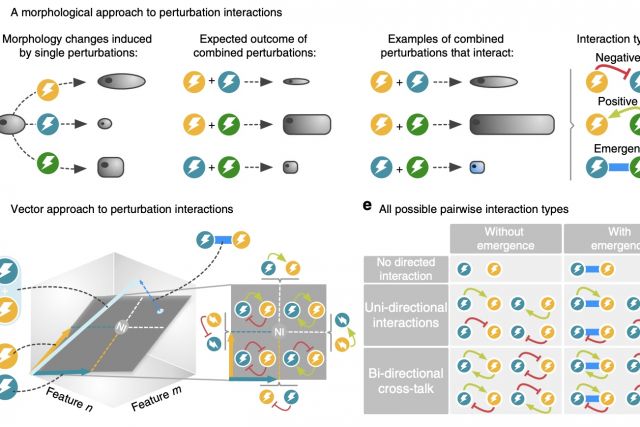
Drug combinations provide effective treatments for diverse diseases, but also represent a major cause of adverse reactions. Currently there is no systematic understanding of how the complex cellular perturbations induced by different drugs influence each other. Here, we introduce a mathematical framework for classifying any interaction between perturbations with high-dimensional effects into 12 interaction types. We apply our framework to a large- scale imaging screen of cell morphology changes induced by diverse drugs and their com- bination, resulting in a perturbome network of 242 drugs and 1832 interactions. Our analysis of the chemical and biological features of the drugs reveals distinct molecular fingerprints for each interaction type. We find a direct link between drug similarities on the cell morphology level and the distance of their respective protein targets within the cellular interactome of molecular interactions. The interactome distance is also predictive for different types of drug interactions.
NATURE COMMUNICATIONS (2019)
doi.org/10.1038/s41467-019-13058-9 [pdf]
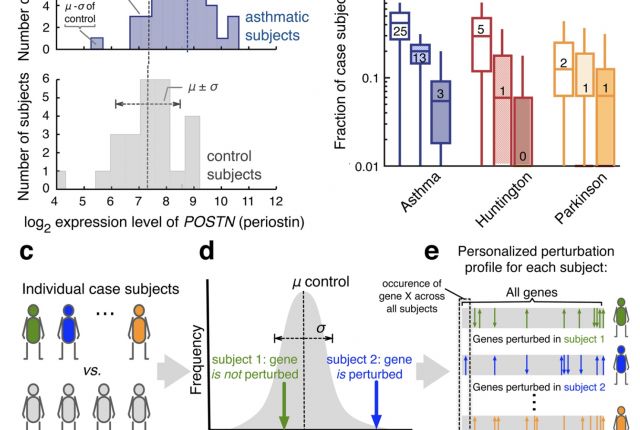
Gene expression data are routinely used to identify genes that on average exhibit different expression levels between a case and a control group. Yet, very few of such differentially expressed genes are detectably perturbed in individual patients. Here, we develop a framework to construct personalized perturbation profiles for individual subjects, identifying the set of genes that are significantly perturbed in each individual. This allows us to characterize the heterogeneity of the molecular manifestations of complex diseases by quantifying the expression-level similarities and differences among patients with the same phenotype. We show that despite the high heterogeneity of the individual perturbation profiles, patients with asthma, Parkinson and Huntington’s disease share a broadpool of sporadically disease-associated genes, and that individuals with statistically significant overlap with this pool have a 80–100% chance of being diagnosed with the disease. The developed framework opens up the possibility to apply gene expression data in the context of precision medicine, with important implications for biomarker identification, drug development, diagnosis and treatment.
npj Systems Biology and Applications (2017)
doi.org/10.1038/s41540-017-0009-0 [pdf]
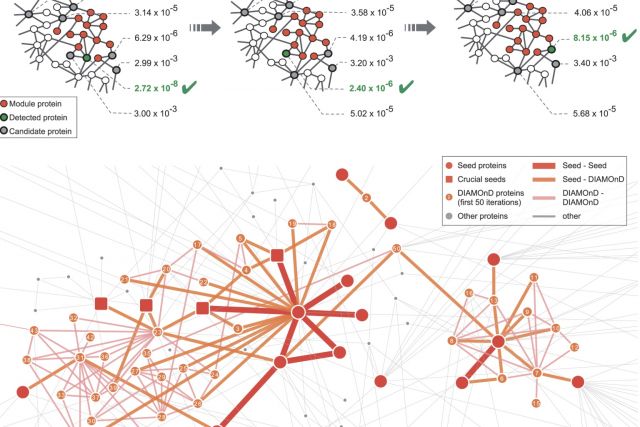
The observation that disease associated proteins often interact with each other has fueled the development of network-based approaches to elucidate the molecular mechanisms of human disease. Such approaches build on the assumption that protein interaction networks can be viewed as maps in which diseases can be identified with localized perturbation within a certain neighborhood. The identification of these neighborhoods, or disease modules, is therefore a prerequisite of a detailed investigation of a particular pathophenotype. While numerous heuristic methods exist that successfully pinpoint disease associated modules, the basic underlying connectivity patterns remain largely unexplored. In this work we aim to fill this gap by analyzing the network properties of a comprehensive corpus of 70 complex diseases. We find that disease associated proteins do not reside within locally dense communities and instead identify connectivity significance as the most predictive quantity. This quantity inspires the design of a novel Disease Module Detection (DIAMOnD) algorithm to identify the full disease module around a set of known disease proteins. We study the performance of the algorithm using well-controlled synthetic data and systematically validate the identified neighborhoods for a large corpus of diseases.
PLOS COMPUTATIONAL BIOLOGY (2017)
doi.org/10.1371/journal.pcbi.1004120 [pdf]
Overview
The human body contains myriads of components that range from biomolecules to cells, tissues and organs. Yet, we are far more than the sum of our parts. The broad ambition of our research is to help us understand exactly how.
The key ingredient for the transition from individual components to collective function, from simple to complex, is the way in which the components interact with each other. We use tools and concepts from network theory to characterize these interaction patterns and interpret their biological implications. A particular focus of our work is the network of all interactions between human proteins. In analogy to the genome, the integrated protein interaction network is also termed interactome. Where the genome provides a blueprint for the individual components of the human body, the interactome provides a blueprint for the collective functions that emerge from their interactions.
Our projects address diverse questions ranging from fundamental organizational principles in biology to practical challenges in medicine. The vignettes below provide several examples, a complete list of publications can be found here.
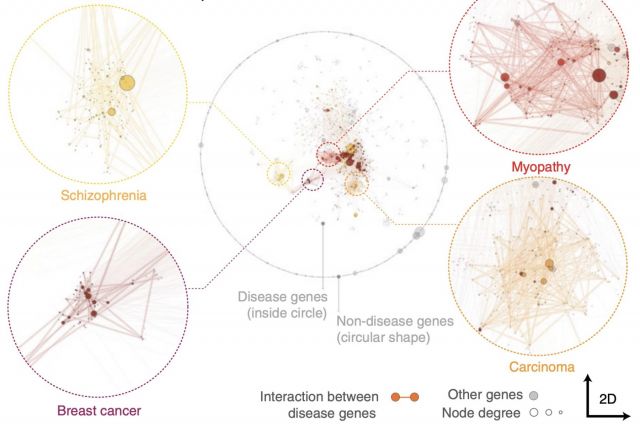
Networks offer an intuitive visual representation of complex systems. Important network characteristics can often be recognized by eye and, in turn, patterns that stand out visually often have a meaningful interpretation. In conventional network layout algorithms, however, the precise determinants of a node’s position within a layout are difficult to decipher and to control. Here we propose an approach for directly encoding arbitrary structural or functional network characteristics into node positions. We introduce a series of two- and three-dimensional layouts, benchmark their efficiency for model networks, and demonstrate their power for elucidating structure-to-function relationships in large-scale biological networks.
NATURE COMPUTATIONAL SCIENCE (2022)
doi.org/10.1038/s43588-022-00199-z [pdf]
NATURE RESEARCH BRIEFING (2022)
doi.org/10.1038/s43588-022-00203-6 [pdf]
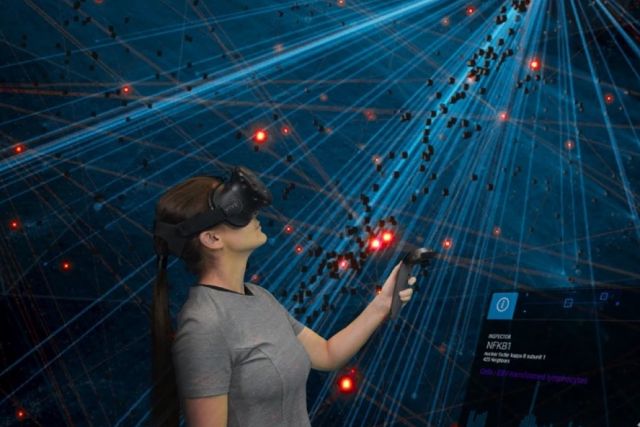
Networks provide a powerful representation of interacting components within complex systems, making them ideal for visually and analytically exploring big data. However, the size and complexity of many networks render static visualizations on typically-sized paper or screens impractical, resulting in proverbial ‘hairballs’. Here, we introduce a Virtual Reality (VR) platform that overcomes these limitations by facilitating the thorough visual, and interactive, exploration of large networks. Our platform allows maximal customization and extendibility, through the import of custom code for data analysis, integration of external databases, and design of arbitrary user interface elements, among other features. As a proof of concept, we show how our platform can be used to interactively explore genome-scale molecular networks to identify genes associated with rare diseases and understand how they might contribute to disease development. Our platform represents a general purpose, VR-based data exploration platform for large and diverse data types by providing an interface that facilitates the interaction between human intuition and state-of-the-art analysis methods.
NATURE COMMUNICATIONS (2021)
doi.org/10.1038/s41467-021-22570-w [pdf]
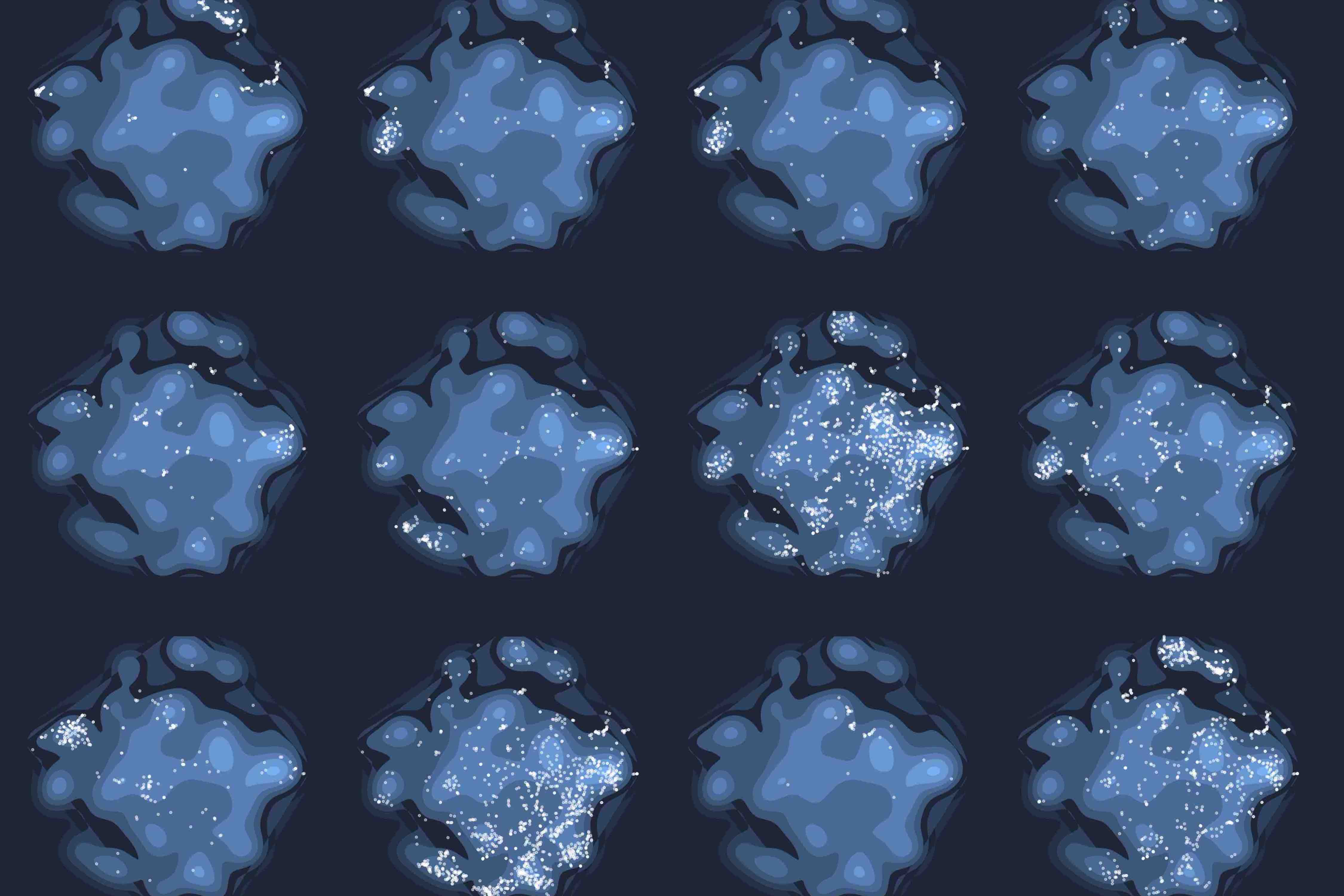
Rare genetic diseases are typically caused by a single gene defect. Despite this clear causal relationship between genotype and phenotype, identifying the pathobiological mechanisms at various levels of biological organization remains a practical and conceptual challenge. Here, we introduce a network approach for evaluating the impact of rare gene defects across biological scales. We construct a multiplex network consisting of over 20 million gene relationships that are organized into 46 network layers spanning six major biological scales between genotype and phenotype. A comprehensive analysis of 3,771 rare diseases reveals distinct phenotypic modules within individual layers. These modules can be exploited to mechanistically dissect the impact of gene defects and accurately predict rare disease gene candidates. Our results show that the disease module formalism can be applied to rare diseases and generalized beyond physical interaction networks. These findings open up new venues to apply network-based tools for cross-scale data integration.
NATURE COMMUNICATIONS (2021)
doi.org/10.1038/s41467-021-26674-1 [pdf]
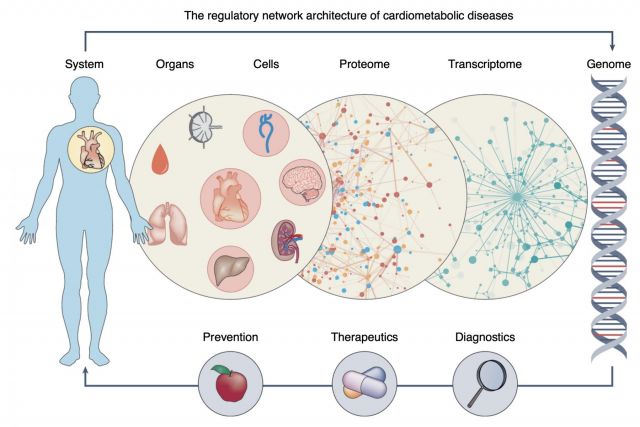
Complex disease definitions often represent descriptive umbrella terms of symptoms rather than mechanistic entities. In this brief News & Views article we highlight a new study that shows how network-based approaches can help identify the mechanisms that link genes, cells, tissues and organs in cardiovascular diseases. We discuss how network medicine approaches can help accelerate the development of early and individualized diagnostics and therapeutics in the coming era of precision medicine.
NATURE GENETICS (2022)
doi.org/10.1038/s41588-021-00994-w [pdf]
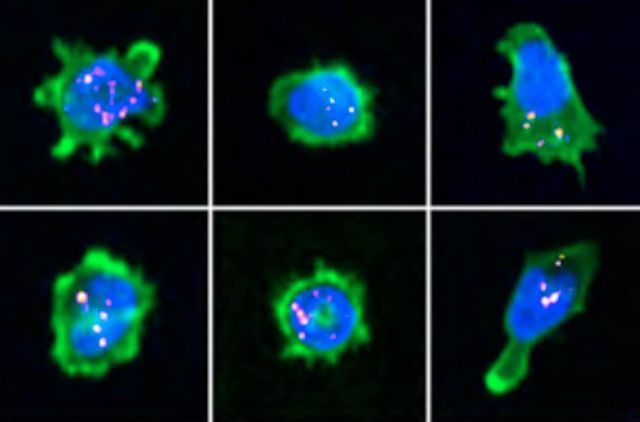
The immunological synapse is a complex structure that decodes stimulatory signals into adapted lympho- cyte responses. It is a unique window to monitor lymphocyte activity because of development of systematic quantitative approaches. Here we demonstrate the applicability of high-content imaging to human T and nat- ural killer (NK) cells and develop a pipeline for unbiased analysis of high-definition morphological profiles. Our approach reveals how distinct facets of actin cytoskeleton remodeling shape immunological synapse archi- tecture and affect lytic granule positioning. Morphological profiling of CD8+ T cells from immunodeficient in- dividuals allows discrimination of the roles of the ARP2/3 subunit ARPC1B and the ARP2/3 activator Wiskott- Aldrich syndrome protein (WASP) in immunological synapse assembly. Single-cell analysis further identifies uncoupling of lytic granules and F-actin radial distribution in ARPC1B-deficient lymphocytes. Our study pro- vides a foundation for development of morphological profiling as a scalable approach to monitor primary lymphocyte responsiveness and to identify complex aspects of lymphocyte micro-architecture.
CELL REPORTS (2021)
doi.org/10.1016/j.celrep.2021.109318 [pdf]
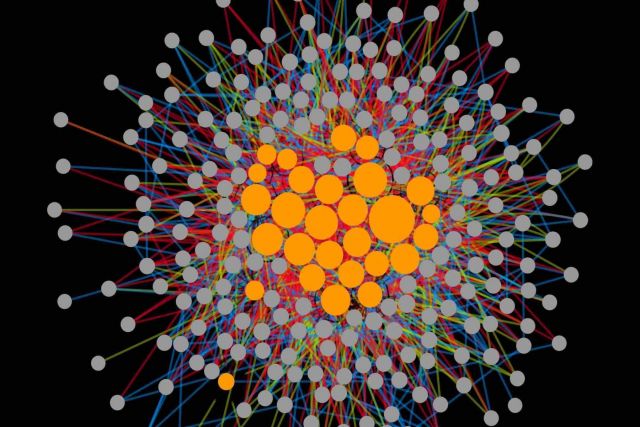
Drug combinations provide effective treatments for diverse diseases, but also represent a major cause of adverse reactions. Currently there is no systematic understanding of how the complex cellular perturbations induced by different drugs influence each other. Here, we introduce a mathematical framework for classifying any interaction between perturbations with high-dimensional effects into 12 interaction types. We apply our framework to a large- scale imaging screen of cell morphology changes induced by diverse drugs and their com- bination, resulting in a perturbome network of 242 drugs and 1832 interactions. Our analysis of the chemical and biological features of the drugs reveals distinct molecular fingerprints for each interaction type. We find a direct link between drug similarities on the cell morphology level and the distance of their respective protein targets within the cellular interactome of molecular interactions. The interactome distance is also predictive for different types of drug interactions.
NATURE COMMUNICATIONS (2019)
doi.org/10.1038/s41467-019-13058-9 [pdf]
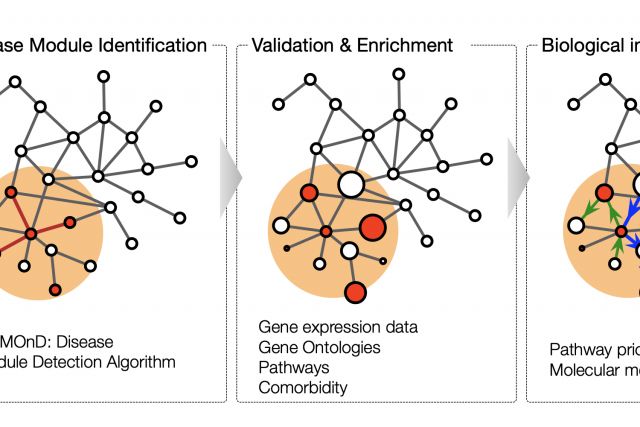
This book chapter aims to give a general introduction to the dynamic field of network medicine. We start with a broad overview of major network types that are relevant to medicine. We then discuss with more detail the cellular network of molecular interactions among proteins and other biomolecules, the perhaps most widely used network in biomedical research. In the last section, we introduce disease module analysis, an important application of network tools to elucidate the molecular mechanisms of a particular disease..
ANALYZING NETWORK DATA IN BIOLOGY AND MEDICINE (2019)
Cambridge University Press [pdf]
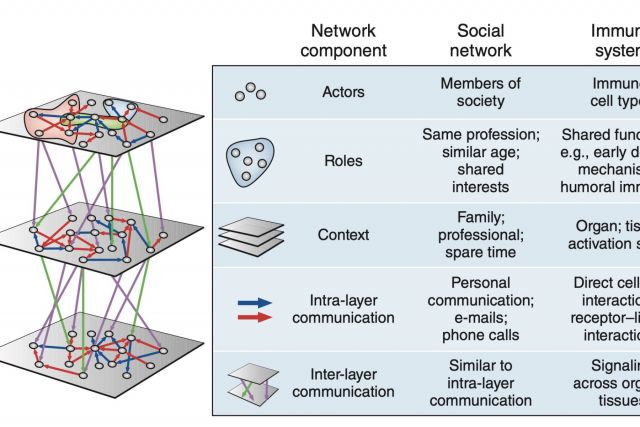
The immune system employs a multitude of molecules, cells and organs that act together throughout the entire body to guard human health. Much like in a social network, immune cells can exert full functionality only through effective collaboration and communication. In this brief News & Views article we highlight a publication by Rieckmann and colleagues presenting a map of this interplay that is unprecedented both in scale and level of detail. We discuss the analogy between the immune systems and a social network.
NATURE IMMUNOLOGY (2017)
doi.org/10.1038/ni.3727 [pdf]
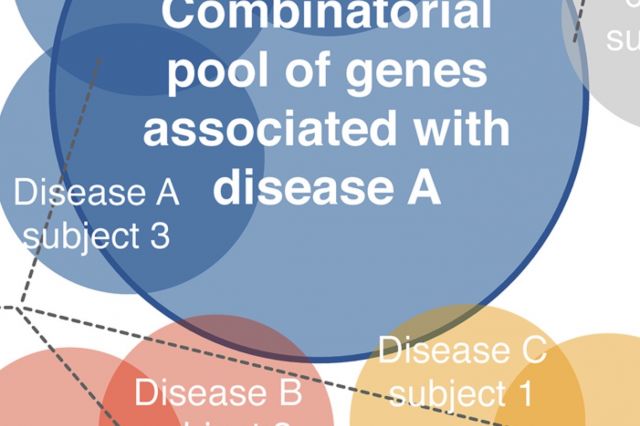
Gene expression data are routinely used to identify genes that on average exhibit different expression levels between a case and a control group. Yet, very few of such differentially expressed genes are detectably perturbed in individual patients. Here, we develop a framework to construct personalized perturbation profiles for individual subjects, identifying the set of genes that are significantly perturbed in each individual. The developed framework opens up the possibility to apply gene expression data in the context of precision medicine, with important implications for biomarker identification, drug development, diagnosis and treatment.
npj Systems Biology and Applications (2017)
doi.org/10.1038/s41540-017-0009-0 [pdf]
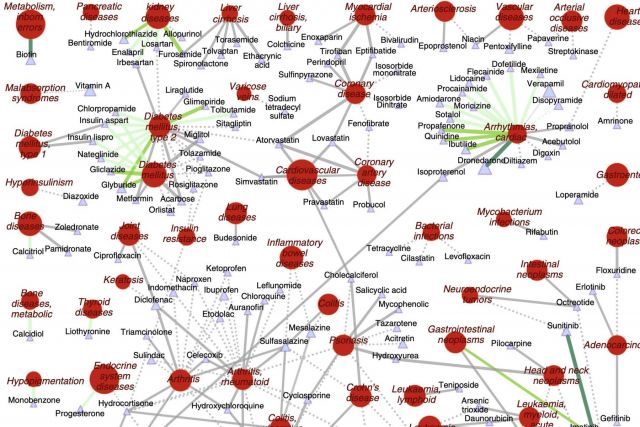
The increasing cost of drug development together with a significant drop in the number of new drug approvals raises the need for innovative approaches for target identification and efficacy prediction. Here, we take advantage of our increasing understanding of the network-based origins of diseases to introduce a drug-disease proximity measure that quantifies the interplay between drugs targets and diseases. By correcting for the known biases of the interactome, proximity helps us uncover the therapeutic effect of drugs, as well as to distinguish palliative from effective treatments. Our analysis of 238 drugs used in 78 diseases indicates that the therapeutic effect of drugs is localized in a small network neighborhood of the disease genes and highlights efficacy issues for drugs used in Parkinson and several inflammatory disorders. Finally, network-based proximity allows us to predict novel drug-disease associations that offer unprecedented opportunities for drug repurposing and the detection of adverse effects.
NATURE COMMUNICATIONS (2016)
doi.org/10.1038/ncomms10331 [pdf]
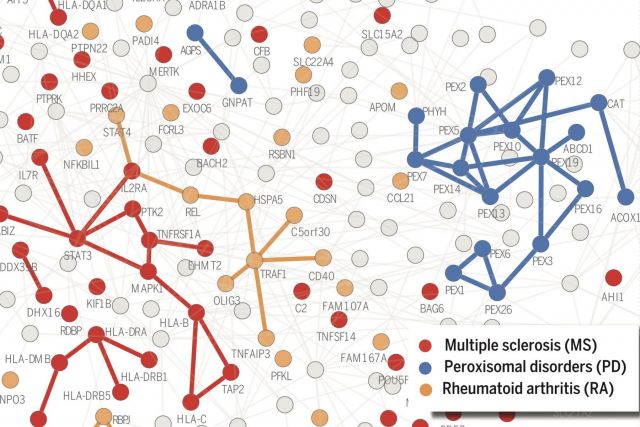
According to the disease module hypothesis, the cellular components associated with a disease segregate in the same neighborhood of the human interactome, the map of biologically relevant molecular interactions. Yet, given the incompleteness of the interactome and the limited knowledge of disease-associated genes, it is not obvious if the available data have sufficient coverage to map out modules associated with each disease. Here we derive mathematical conditions for the identifiability of disease modules and show that the network-based location of each disease module determines its pathobiological relationship to other diseases. For example, diseases with overlapping network modules show significant coexpression patterns, symptom similarity, and comorbidity, whereas diseases residing in separated network neighborhoods are phenotypically distinct. These tools represent an interactome-based platform to predict molecular commonalities between phenotypically related diseases, even if they do not share primary disease genes.
SCIENCE (2015)
doi.org/10.1126/science.1257601 [pdf]
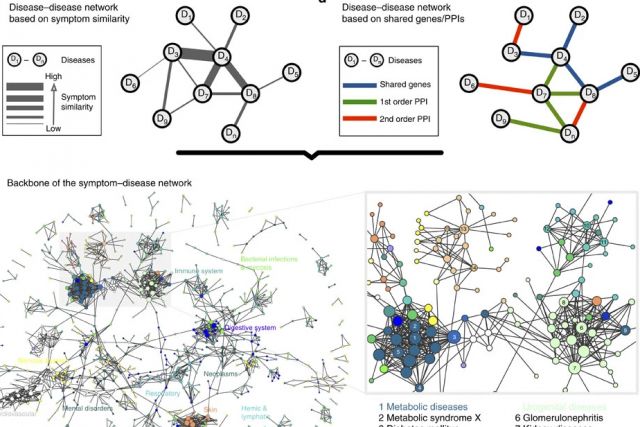
In the post-genomic era, the elucidation of the relationship between the molecular origins of diseases and their resulting phenotypes is a crucial task for medical research. Here, we use a large-scale biomedical literature database to construct a symptom-based human disease network and investigate the connection between clinical manifestations of diseases and their underlying molecular interactions. We find that the symptom-based similarity of two diseases correlates strongly with the number of shared genetic associations and the extent to which their associated proteins interact. Moreover, the diversity of the clinical manifestations of a disease can be related to the connectivity patterns of the underlying protein interaction network. The comprehensive, high-quality map of disease–symptom relations can further be used as a resource helping to address important questions in the field of systems medicine, for example, the identification of unexpected associations between diseases, disease etiology research or drug design.
NATURE COMMUNICATIONS (2014)
doi.org/10.1038/ncomms5212 [pdf]